Blog
The Ethical Landscape of Data Science and Analytics
- July 24, 2023
- Posted by: Oyesh
- Category: Technology
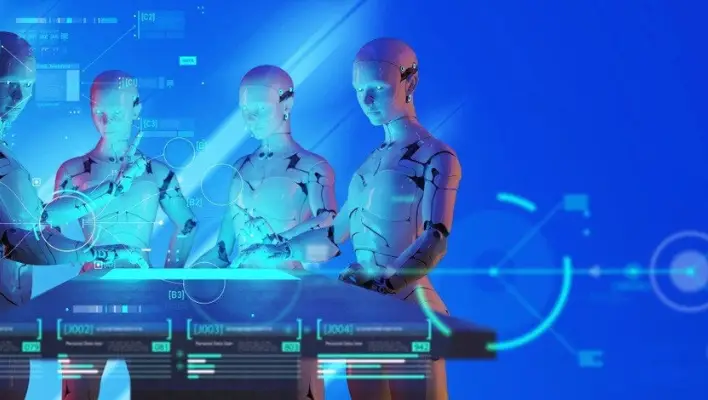
Introduction
In our technologically driven world, there’s no denying the pervasive influence of Data Science and Data Analytics. These fields have become vital tools for businesses worldwide, providing valuable insights and guiding strategic decisions. However, with this power comes a great responsibility. As we harness the potential of these data-driven disciplines, the ethical landscape of data science and data analytics is increasingly coming under scrutiny.
Understanding Data Science and Data Analytics
Before diving into the ethical landscape, it is important to grasp the significance of Data Science and Data Analytics.
What is Data Science?
Data Science is a multidisciplinary field that utilizes scientific methods, processes, algorithms, and systems to extract knowledge and insights from structured and unstructured data. It amalgamates statistics, data analysis, and machine learning to analyze and interpret complex phenomena for decision-making.
What is Data Analytics?
Data Analytics is the science of analyzing raw data to make conclusions about that information. It involves several techniques and processes aimed at enhancing productivity and business gain. Data Analytics is segmented into four types – Descriptive, Diagnostic, Predictive, and Prescriptive Analytics.
While both fields are distinct, they share a common goal: to glean valuable insights from data that can drive strategic decisions and actions.
The Emergence of the Ethical Landscape
As the potential and application of data science and data analytics expand, so do ethical challenges. The ethical landscape of data science and data analytics is multifaceted, dealing with issues such as data privacy, bias in algorithms, and transparency of methods.
Data Privacy
In the world of data science and analytics, data privacy has taken center stage. Businesses are gathering vast amounts of data, often without explicit consent from individuals. The question of who owns this data, how it should be stored, and who can access it is a vital ethical concern.
Algorithmic Bias
Algorithmic bias refers to systematic and repeatable errors in a computer system that produce unfair outcomes. Bias can creep in through the data used to train the model, the design of the algorithm, or the intentions of those using it. Regardless of the source, it can lead to discriminatory practices and unfair outcomes.
Transparency
Transparency is another critical concern in the ethical landscape of data science and analytics. As data scientists develop increasingly complex algorithms, understanding how these algorithms make decisions can become difficult. Without clear explanations, users may feel uneasy about the technology, leading to mistrust and resistance.
Navigating the Ethical Landscape
Understanding the ethical landscape of data science and data analytics is the first step. The next is to navigate these challenges effectively, ensuring data is used responsibly and ethically.
Ethical Guidelines
The development and implementation of ethical guidelines can serve as a roadmap for data scientists and analysts. These guidelines should outline how to handle data, how to address potential bias, and how to maintain transparency.
Legal Regulations
Legal regulations are another crucial tool in navigating the ethical landscape. They provide a framework for data handling and privacy, and enforce consequences for those who violate these standards. Examples include the General Data Protection Regulation (GDPR) in the EU and the California Consumer Privacy Act (CCPA) in the US.
Education and Training
Finally, education and training play a significant role in ethical data science and data analytics. By teaching data scientists and analysts about the potential ethical implications of their work, they can be better prepared to make ethical decisions and create fair and unbiased algorithms.
Deep Dive into Ethical Issues in Data Science and Analytics
Now that we’ve touched upon the broad contours of the ethical landscape, let’s delve deeper into the key ethical issues in data science and data analytics.
Data Misuse
One of the primary ethical issues revolves around data misuse. With the wealth of data available, the potential for misuse is high. This can range from unauthorized access to personal data, through data breaches, to using data in ways that were not initially consented to by the data subject. It’s crucial that organizations have strict data usage policies and security measures to prevent such occurrences.
Informed Consent
Closely related to data misuse is the concept of informed consent. This pertains to the idea that data subjects should be fully aware of how their data will be used before it is collected. However, in practice, obtaining informed consent is often challenging due to vague or complicated privacy policies that can be difficult for users to understand.
Data Quality
Data quality is another ethical issue that data scientists and analysts grapple with. Poor data quality can lead to inaccurate insights, faulty decision-making, and potential harm to individuals or groups. Ensuring data is accurate, reliable, and consistent is an ethical responsibility that must not be taken lightly.
Black Box Algorithms
The issue of ‘black box’ algorithms is another serious ethical concern. These are complex algorithms that offer little to no insight into how they make their decisions. When used in critical areas like healthcare or criminal justice, these algorithms can have profound and sometimes detrimental effects on people’s lives.
Case Studies Highlighting Ethical Issues
To better comprehend the ethical landscape of data science and analytics, it’s worthwhile to examine some real-world examples.
The Facebook-Cambridge Analytica Scandal
In 2018, news broke that political consulting firm Cambridge Analytica had harvested the personal data of millions of people’s Facebook profiles without their consent and used it for political advertising purposes. This incident brought the issues of data privacy and informed consent into the global spotlight, highlighting the potential for misuse of data and the need for tighter regulations.
Amazon’s Gender-Biased Recruitment Algorithm
In 2014, Amazon discovered that their AI recruiting tool was not gender-neutral. The algorithm favored male candidates over females for technical job roles because it was trained on resumes submitted to the company over a ten-year period, most of which came from men. This case underscores the potential for bias in algorithms and the need for careful oversight in their development.
Developing an Ethical Framework for Data Science and Analytics
Given the myriad of ethical issues surrounding data science and analytics, developing an ethical framework is paramount. This framework should provide clear guidelines for data collection, usage, storage, and disposal, and ensure transparency and fairness in data processing.
Data Stewardship
Data stewardship is a key component of any ethical framework. It encompasses the management and oversight of an organization’s data assets to provide business users with high-quality data that is easy to access in a consistent manner. Good data stewardship can help prevent data misuse and ensure data quality.
Algorithmic Accountability
Algorithmic accountability is another cornerstone of an ethical framework. It involves making sure that algorithms are transparent, understandable, and accountable for their decisions. Techniques such as Explainable AI (XAI), which aim to make the decision-making process of AI systems clear and understandable to human users, can help achieve this.
Privacy by Design
Privacy by Design is a principle that calls for privacy to be taken into account throughout the whole engineering process. It involves integrating privacy principles into the design specifications of technologies, business practices, and physical infrastructures. By incorporating this into the ethical framework, organizations can ensure that data privacy is not an afterthought, but a key consideration from the outset.
Embracing Ethical Practices in Data Science and Data Analytics
Embracing ethical practices in data science and data analytics is not just about preventing harm or adhering to regulations. It’s also about building trust with customers and the public. In a world where data breaches and misuse of data are increasingly common, organizations that prioritize ethical practices can differentiate themselves and win the trust of their stakeholders.
Ethical Leadership
Leaders play a crucial role in fostering an ethical culture within an organization. By setting the tone from the top, leaders can ensure that ethical practices are ingrained in the organization’s DNA and are followed at all levels.
Continuous Learning and Improvement
The ethical landscape of data science and analytics is continually evolving. Therefore, organizations must commit to continuous learning and improvement. This involves staying up-to-date with the latest developments, learning from past mistakes, and always striving to do better.
The Future of the Ethical Landscape of Data Science and Analytics
As the field of data science and data analytics continues to grow, the ethical landscape will also evolve. New challenges will emerge, and our understanding of current issues will deepen. But one thing remains clear: the ethical landscape of data science and data analytics will continue to be a critical area of focus in our increasingly data-driven world.
Conclusion
In conclusion, the ethical landscape of data science and data analytics is a complex yet necessary component of these growing fields. As we continue to use data to drive decision-making, it’s imperative to remember the ethical implications of our actions. By acknowledging and addressing these ethical concerns, we can ensure a future where data science and analytics are used for the betterment of society, rather than its detriment.
Frequently Asked Questions
Q: What is the ethical landscape of data science and data analytics?
A: The ethical landscape of data science and data analytics refers to the moral questions and implications surrounding the use and application of data science and analytics. This includes concerns about data privacy, bias in algorithms, misuse of data, informed consent, data quality, and transparency, among others.
Q: Why is data privacy a critical concern in the ethical landscape of data science and data analytics?
A: Data privacy is a crucial concern because of the vast amounts of personal and sensitive data that organizations collect. The question of who owns this data, how it should be stored, and who can access it, is vital for protecting individual rights and freedoms. Misuse of this data can lead to violations of privacy and even harm to individuals or groups.
Q: How does algorithmic bias pose an ethical challenge in data science and analytics?
A: Algorithmic bias occurs when systematic errors in a computer system produce unfair outcomes. These biases can creep into algorithms through the data used to train them, their design, or the intentions of those using them. Biased algorithms can result in discriminatory practices and unfair outcomes, which makes it a critical ethical challenge.
Q: What are some ways to navigate the ethical landscape of data science and analytics?
A: Navigating the ethical landscape involves the development of ethical guidelines for data scientists and analysts, the establishment of legal regulations to provide a framework for data handling and privacy, and education and training for practitioners. Additionally, fostering ethical leadership and promoting continuous learning and improvement within organizations are also key.
Q: How does the ethical landscape of data science and data analytics impact organizations?
A: The ethical landscape impacts organizations in numerous ways. Ethical mishaps can lead to legal consequences, financial loss, and damage to an organization’s reputation. On the other hand, organizations that prioritize ethical practices in data science and data analytics can build trust with customers and differentiate themselves in the market.