Blog
Data Science 2030: Key Trends & Tech Transforming the Industry
- April 26, 2023
- Posted by: Vidal International
- Category: Technology
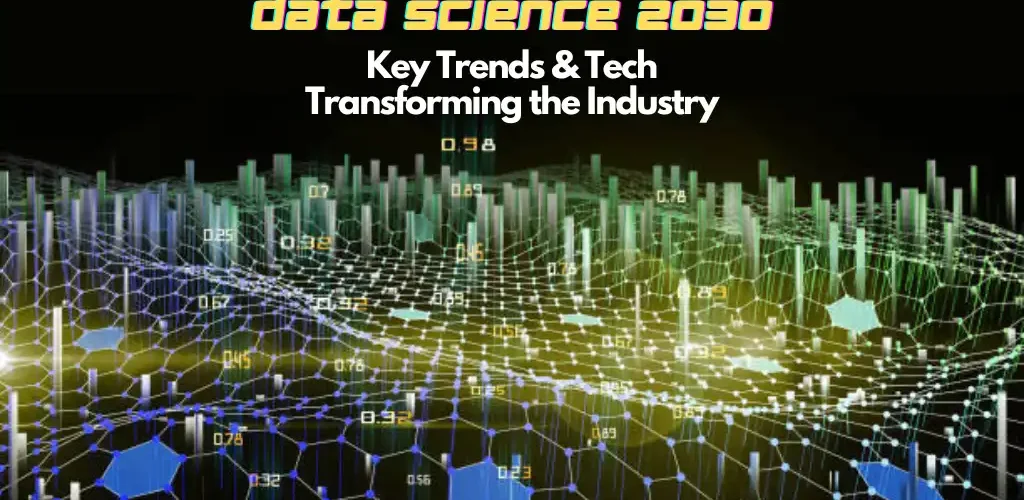
Introduction
As we venture into the future, the data science landscape rapidly evolves. By 2030, several groundbreaking trends and technologies will have transformed the industry. In this article, we will discuss the key trends and technologies revolutionizing the data science 2030 landscape and shaping the future of this dynamic field.
Trend 1: Artificial Intelligence and Machine Learning
Deep Learning and Neural Networks
Artificial intelligence (AI) and machine learning (ML) are central to the advancements in data science. As algorithms become more sophisticated, AI and ML technologies enable data scientists to analyze complex data sets and uncover previously hidden insights. One of the key aspects of AI and ML is deep learning, which leverages artificial neural networks to learn complex patterns and make accurate predictions. By 2030, deep learning and other AI technologies will be fully integrated into most aspects of data science, including data preparation, analysis, visualization, and interpretation.
Reinforcement Learning for Data Science 2030
Another promising area in AI and ML is reinforcement learning, which focuses on training algorithms to learn from their environment and make feedback-based decisions. Reinforcement learning has already shown great potential in various applications, such as robotics, gaming, and recommendation systems. By 2030, reinforcement learning techniques will likely be widely adopted in data science, enabling more advanced decision-making and optimization processes.
Trend 2: Automation in Data Science
Automated Feature Engineering
Automation is another significant trend shaping the data science industry. As data volumes grow, automation technologies will be crucial in streamlining the data science process. One aspect of automation in data science is automated feature engineering, which involves using machine learning algorithms to identify and create relevant features from raw data automatically. This process can significantly reduce the time and effort required for manual feature engineering, allowing data scientists to focus on more strategic aspects of their work.
Automated Model Selection
Another aspect of automation in data science is automated model selection. This involves using AI and ML algorithms to automatically identify the best model for a given dataset and problem. Automating the model selection process allows data scientists to avoid spending countless hours testing and tuning various models, leading to more efficient and accurate results. By 2030, we expect the widespread adoption of automation tools and technologies to improve efficiency and productivity in data science significantly.
Trend 3: Edge Computing and IoT
Real-time Data Processing
The emergence of edge computing and the Internet of Things (IoT) will significantly impact data science. With billions of IoT devices generating massive amounts of data, edge computing will enable real-time processing and analysis of this data closer to its source. This will result in reduced latency, improved efficiency, and enhanced decision-making capabilities.
Smart Devices and Their Impact
The proliferation of intelligent devices, such as wearables, smart home appliances, and industrial sensors, has led to an explosion of data that needs to be processed and analyzed. By 2030, we foresee edge computing and IoT becoming indispensable components of the data science ecosystem, driving innovation in real-time analytics, predictive maintenance, and personalized recommendations.
Trend 4: Quantum Computing and its Impact
Quantum Algorithms
Quantum computing has the potential to revolutionize the data science landscape by enabling unprecedented processing power. Quantum computers can solve complex optimization problems and perform calculations at orders of magnitude faster than traditional computers. By 2030, quantum computing is expected to significantly impact data science by enabling new ways of processing and analyzing data and solving previously unsolvable problems.
Challenges and Opportunities
Despite its immense potential, quantum computing also presents several challenges that must be overcome before fully integrating into the data science landscape. These challenges include developing scalable quantum hardware, creating efficient quantum algorithms, and addressing error correction and stability issues. However, by 2030, we expect significant progress in addressing these challenges, leading to new opportunities and applications in data science.
Trend 5: Data Privacy and Security
GDPR and Other Regulations
As data science advances, so will concerns surrounding data privacy and security. By 2030, data privacy regulations and security measures will become even more stringent, with global regulations such as the European Union’s General Data Protection Regulation (GDPR) setting the standard for data protection. Organizations must adapt their data practices to comply with these regulations, ensuring that personal information is processed and stored securely.
Privacy-Preserving Techniques
In addition to regulatory compliance, data scientists will increasingly need to adopt privacy-preserving techniques to protect sensitive data. These techniques, such as differential privacy and homomorphic encryption, enable data analysis while minimizing the risk of exposing personal information. By 2030, we expect privacy-preserving techniques to become a critical component of data science workflows, ensuring that sensitive data is protected and privacy concerns are addressed.
Trend 6: The Rise of Augmented Analytics
Natural Language Processing (NLP)
Augmented analytics represents the next wave of innovation in data science, combining AI, ML, and natural language processing (NLP) to enhance human decision-making capabilities. NLP, in particular, will enable data scientists to interact with their data using natural language queries, making it easier to discover new insights and make data-driven decisions more efficiently.
Automatic Insights Generation
Another key aspect of augmented analytics is automatically generating insights from data. Advanced algorithms will analyze data sets, identify patterns, and generate actionable insights without manual intervention. This automation will democratize access to advanced analytics, allowing non-experts to benefit from the power of data science.
Trend 7: The Growing Importance of Data Visualization
Advanced Visualization Tools
Data visualization is becoming an increasingly important aspect of data science, enabling data scientists to communicate their findings and insights to a broader audience effectively. By 2030, data visualization will be a critical skill for data scientists, with advanced visualization tools and techniques being widely adopted across the industry. This trend will lead to more intuitive and interactive visualizations that convey complex data insights.
Storytelling with Data
In addition to advanced visualization tools, effective data storytelling will become a vital skill for data scientists. Data storytelling involves visualizations, narratives, and context to clearly and persuasively communicate data insights. By mastering data storytelling, data scientists will be better equipped to share their findings with technical and non-technical audiences, fostering collaboration and driving data-driven decision-making.
Trend 8: Demand for Skilled Data Science Professionals
Data Science Education and Training
As the field of data science continues to grow and evolve, there will be a significant demand for skilled data science professionals. By 2030, the demand for data scientists, engineers, and related professionals will increase exponentially. This trend highlights the need for continued investment in data science education and training programs to ensure the workforce has the necessary skills and expertise to drive the industry forward.
Future Job Opportunities for Data Science
The growing demand for skilled data science professionals will also create new job opportunities in various industries, ranging from finance and healthcare to retail and transportation. In addition, as organizations increasingly recognize the value of data-driven decision-making, data science professionals will be in high demand to help businesses make sense of their data and transform it into actionable insights.
Conclusion on Data Science 2030
In conclusion, the data science landscape is poised for significant transformation by 2030. The key trends and technologies discussed in this article, such as AI and ML, automation, edge computing and IoT, quantum computing, data privacy and security, augmented analytics, data visualization, and the demand for skilled professionals, will all play a critical role in shaping the future of the industry. By staying informed about these trends and adapting to the changes they bring, data science professionals and organizations can position themselves for success in the years to come.
As we look forward to the advancements and innovations that will define data science in 2030, it is essential for professionals to continuously develop their skills and stay up-to-date with the latest trends and technologies. By doing so, they can contribute to the growth and evolution of this dynamic field, driving the development of new solutions and unlocking the full potential of data-driven decision-making.
As the data science landscape evolves, businesses must understand the impact of these trends and technologies on their operations and adapt their strategies accordingly. By embracing the power of data science and leveraging the latest advancements in AI, ML, and other areas, organizations can gain a competitive edge in the market and drive sustainable growth.
Ultimately, the future of data science lies in our ability to harness the power of data to inform decision-making, improve efficiency, and deliver better outcomes for individuals, businesses, and society. By understanding and embracing the key trends and technologies shaping the industry, we can ensure that data science continues to drive innovation and progress in the years to come.